
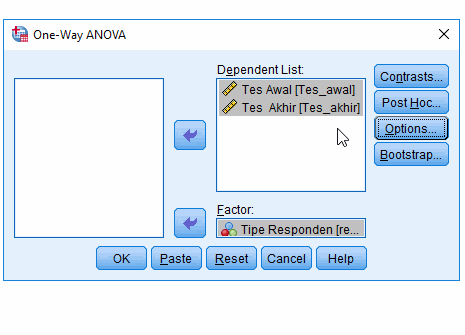
Whether a participant is 20 or 50, the mean satisfaction is around 55. In the control condition and the green condition, age isn’t really related to satisfaction. Okay, what does this mean for the analysis, and more importantly, the conclusions we draw about the data? What the interaction says about the data Quite significant.Ĭlearly, the ANCOVA assumption of parallel lines is not being met. The p-value for the interaction between age and experimental group was. This means that the relationship between age and satisfaction is not the same in every condition. It should be quite clear that the four lines are not parallel. There were no significant differences in the mean age across groups, indicating that random assignment worked well. The vertical black line at Age=25.8 represents the mean age of all participants. (Incidentally, the fact that they all cross at the same age is a coincidence-this doesn’t have to happen). So, for example, you can see that the mean satisfaction score is just about equal in all four groups at the point where the four lines cross, around age 25. The purple line is the control group.Īs they’re regression lines, the height of each line at a specific age can be interpreted as the mean value of satisfaction at that age for that specific group. The colored lines indicate the association between age and satisfaction within each of the four experimental groups. Participants’ age was used as a covariate, as previous research indicated that it is related to satisfaction. The outcome variable is satisfaction with their training experience and each condition implemented a specific learning strategy.
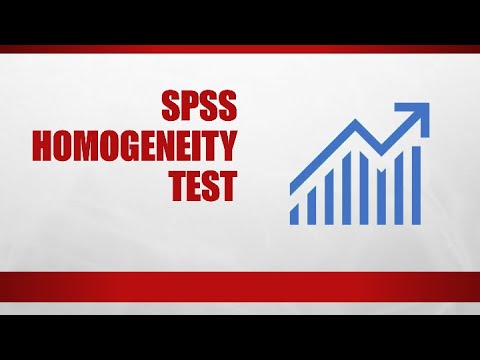
In this (real) example, adult learners were assigned to one of four educational conditions: a control and three experimental conditions. Once again, rather than focus on the “rules”, think about what an interaction tells you about the effects of the independent variable, and how you can best communicate the results. So what does it mean, and what should you do, if you find an interaction between the categorical IV and the continuous covariate? In a previous post, I showed a detailed example for an observational study where the first assumption is irrelevant, but I have gotten a number of questions about the second. There is no interaction between independent variable and the covariate. The independent variable and the covariate are independent of each other.Ģ. There are two oft-cited assumptions for Analysis of Covariance (ANCOVA), which is used to assess the effect of a categorical independent variable on a numerical dependent variable while controlling for a numerical covariate:ġ.
